Unleashing the Hydrogen Energy Revolution: How AI is Powering the Future
Our global reliance on fossil fuels is one of the leading causes of climate change. When fossil fuels are burnt for energy, greenhouse gases and particulate matter are released into the atmosphere. As the hunt for a cleaner energy alternative continues, hydrogen is currently in prime position to help bridge the transition from fossil fuels to greener energy industries.
Compared to fossil fuels, hydrogen gas has several benefits. Firstly, it releases more energy per unit of weight. Secondly and most importantly, it does not release any harmful greenhouse emissions when used as an energy source. Additionally, as it can be produced from water using renewable energy sources like solar and wind power, there is less chance of fuel-based geopolitical conflicts as countries won’t be limited by their own natural resource limits.
Despite all of this, there are still challenges to be addressed before the hydrogen industry can be properly established. To solve these challenges, research scientists are currently leveraging the power of artificial intelligence (AI) and machine learning in a multitude of ways including hydrogen production, storage and distribution.
Storage Materials Discovery
One of the biggest current problems with using hydrogen in the energy industry is the difficulty in storing it. Rather than compressing it into risky high-pressure tanks or cooling it down to a liquid in a very energy-costly process, storing the gas in solid materials has been an exciting promise. However, no suitable material has currently been found that meets all the physical requirements for widespread practical use.
To find the best material, machine learning can be a great tool when combined with high-throughput screening. High throughput screening is a method of testing many samples quickly to assess their properties before trialling out a lead experimentally. By combining it with the pattern recognition abilities of machine learning, it can be significantly more efficient in mapping structure-property relationship, thus accelerating the design of new materials.
Research teams are currently using deep learning (a type of neural network) to train on data of know materials as inputs and hydrogen capacity as outputs. Neural networks are a machine learning tool that was based around the structure of the brain. In simple terms it just follows a series of mathematic transformations across different layers. When the number of layers increase to create a “deeper” network, we call it a deep learning problem. Deep learning is a very effective method for exploring chemical space to find new materials and a brief article on the application of machine learning to the development of new materials with hydrogen storage potential can be found.
Optimising production
Green hydrogen is produced when water is split into hydrogen and oxygen using renewable energy sources like solar and wind power, with zero or little carbon dioxide emission. It is split through process known as electrolysis and can be improved by using AI to make real-time adjustments of electrolysis parameters (e.g. voltage and temperature) using a reinforcement agent. A reinforcement agent is a type of AI that learns by interacting with the environment. It is used in reinforcement learning where an agent makes decisions to maximise cumulative rewards by taking actions and being penalised or rewarded for the actions taken. By using a trained reinforcement model, there can be real-time optimisation of the H2 production processes, making sure that there is the highest possible H2 output at the lowest energy cost.
Forecasting
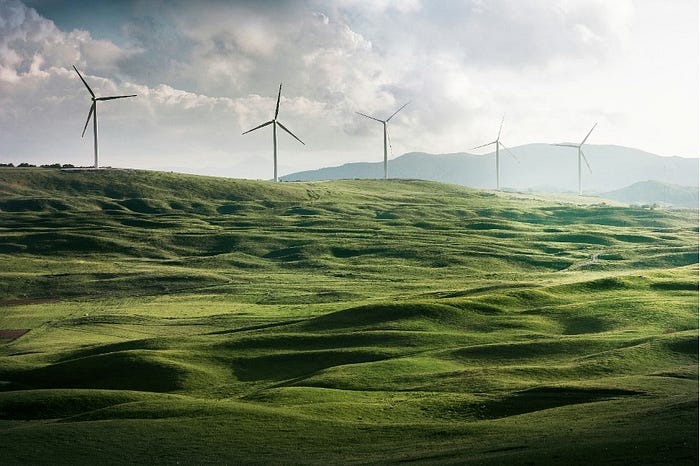
Forecasting is making predictions for future events or outcomes and is an important step in the way that AI can help take the hydrogen industry off the ground. Renewable energy output is variable. For example, some days may be sunnier and have a higher solar energy output than cloudier days. By accurately forecasting renewable energy generation through analysis of data like cloud cover and wind speed, the electrolysis process can be optimised by ramping up the electrolysers based on how the predicted output. A research paper can be found here on the way machine learning forecasting can help in H2 production. The research team used a type of neural network known as a convolution neural network (CNN), which uses small filters to detect basic features before then simplifying it and then combined it to detect more complex pattern.